by Vaios Vaitsis, Founder & CEO at Validata Group
To win, serve and retain customers, testing activities are becoming more pivotal as business process quality is deemed more important than time to market. Beginning with the business-driven change request, through to project scoping and on to DevOps adoption, the infrastructure for technical and regression testing must be present and aligned to every phase.
Improving customer experience is now the highest priority for testers, followed by release on-time and increasing test automation. Testing cannot be an ‘after thought’ and the new business-driven approach to testing is key if organisations are to become ready for continuous delivery of change and ensure that our tests accurately reflect real life production scenarios.
In fact creating testing scenarios that accurately reflect business processes should be the top test planning challenge. Test managers lack visibility into test readiness; in other words they lack confidence that tests reflect real life business scenarios. They lack progress visibility and production data availability for test case creation; therefore they test more and delay delivery. The availability to recreate or duplicate defects is still the pain point with regards to defect management.
For the organisation to become more agile is critical to business and IT to align better and collaborate.
To address the above challenges, we need to choose an end-to-end test automation solution that provides an always up-to-date mirror of production that captures business knowledge of the relevant cases to automate. Create and maintain automated test cases that are based on real-life production data. Business domain expertise is key requirement for testers if they were to adapt agile and continuous delivery.
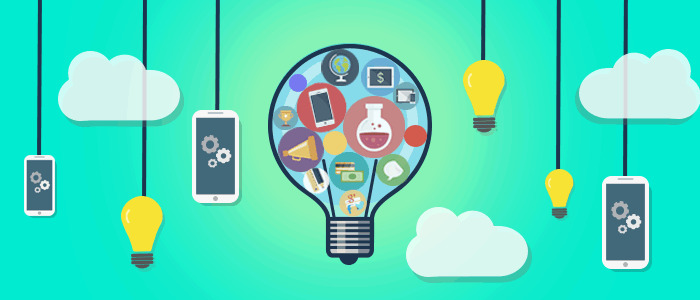
Validating business process integrity rather than just functional features is what business model-driven testing is all about. To achieve this, we need business oriented testing reports which are easily understood by business people. Visibility into the impact of defects on business process workflows and test analytics on root cause context will enable us to understand consequences and prioritise resources. Leveraging machine learning to predict defects will make our testing decisions better and will increase speed and quality.
Machine learning and AI can be used for test management, analysing data from past projects to drive the current testing efforts to the right direction. For example mining defect data from past releases and then exploiting this data to define test suites which find defects and application areas most prone to defects to improve your organization's testing practices.
With banks turning more and more to digital and mobile banking, ensuring that enhanced user experience and seamless transactions are being provided is a key to success. Testing a mobile app eliminates the cost of mobile app failure and poor user experiences. The need for cross channel and cross browser coverage leads financial services organisations and banks leverage intelligent automation, analytics and AI test management to achieve digital transformation.
Testing must be transformed into a continuous and efficient end-to-end quality engineering function, able to run tests in parallel across a wide selection of operating systems, browsers, and resolutions and leveraging the power of the cloud and SaaS models for cost optimisation and better resource planning. Cloud and SaaS are the enablers for the Digital Transformation allowing organizations to adapt and use their IT and software architecture almost instantaneously on an as-needed basis.
Digital Transformation is not just about using new digital technologies to become better or faster; but also focusing on applying these technologies to transform customer experience and deliver customer value.
Related items
- Modernizing Reconciliation and Investigation for Payment operations
- Transforming Payment Reconciliations and Investigations for improved operational efficiency
- 2023's Top Banking Software Glitches Exposed
- Leveraging Synthetic Test Data for Software Testing
- Revolutionizing Reconciliations with Artificial Intelligence