Getting Quality Performance right is key to the success of every area of the QA business. Organisations need to see results across projects, departments and geographies in order to make faster and more informed decisions.
In software projects, it is most important to measure the quality, cost and effectiveness of the project and the processes. To do that, test managers need to define the right metrics as they refine their testing best practices and adopt innovative new methods. The questions that a Test Manager usually asks about his project include:
- Are the sprints on track?
- Will we release on time?
- Do we fix defects fast enough?
- What are the burn down charts telling me?
That has been adequate so far, but with new technologies emerging that employ artificial intelligence (AI) techniques, such as machine learning, a test manager can now go deeper and be able to make more intelligent testing decisions. Machine learning actually helps in getting answers to questions like:
- How many tests have we executed today? How many more tests are we going to need to run?
- How many defects are we going to find? Are we opening defects faster than closing them?
- How long has a defect been in a particular status? What is the ratio of defect closure? Is defect fix ratio acceptable?
- Can I quickly assess the complexity and cost of the project in real-time?
And this because it allows you to examine data from a much wider range of scenarios that affect the quality of your deliverables.
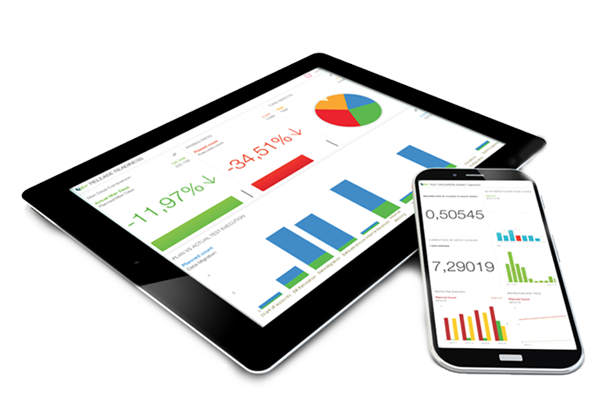
Validata360° platform uses prediction models based on extensive data mining and iterative learning techniques that maximise the efficiency and minimises costs and risks related to recurring software lifecycles such as:
Defect Resolution and Defect Finding Velocity
This model provides a 360 view of the defect management consolidating data from multiple projects. Mapping defect trends across projects and products enables test and project managers to manage their resources better to keep the delivery on schedule.
Release Readiness
This is a dynamic model that provides insights on the progress and quality of test design and execution coverage against requirements. Higher test automation coverage ensures that efficiency is improved and costs are reduced and that you are getting the maximum gains from the automation.
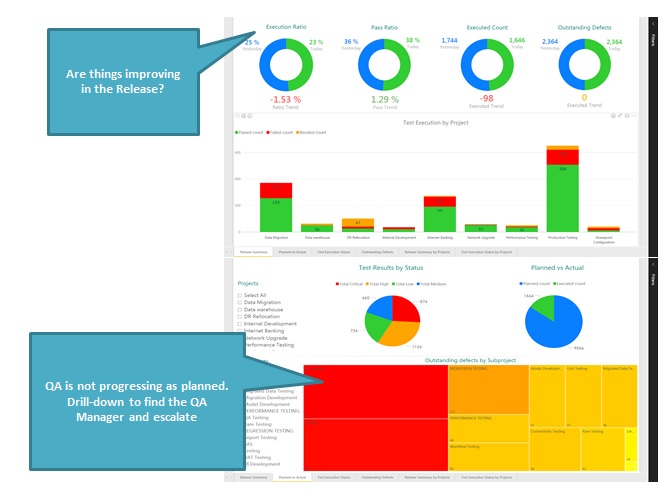
These predictive models power Validata360° to help automate the risk analysis and test management that sit at the heart of software quality management.
Tagged under
Related items
- The Evolution of Payment Data: Why ISO 20022 Conversion Is a Game-Changer
- Modernizing Reconciliation and Investigation for Payment operations
- Transforming Payment Reconciliations and Investigations for improved operational efficiency
- 2023's Top Banking Software Glitches Exposed
- Breaking Free: Why It's Time to Ditch Manual Spreadsheets for Reconciliation