Can you imagine being able to mine every bit of value possible from your datasets? If not, it’s because the privacy fears and data leaks risks put decision makers in financial sector in a fake dilemma: Protecting the privacy of your customers and keeping their sensitive data secure or getting agile through privacy innovation? Is there a way to get the best of both sides without sacrificing on privacy and security?
Synthetic data bridges the gap between security and innovation making at the same time good use of the expertise, reliability and swatches of valuable data that banks bring to the table combined with the agility, creativity and a fresh perspective that fintechs offer.
It’s true that in the financial sector you can’t afford to play fast and loose with sensitive data, but the good news is that there is actually a way to keep up with the pace of digital transformation, at a time when market trends are dynamic and unpredictable. For example, synthetic data for financial services enables banks and other financial institutions to build models that vastly improve KYC and customer onboarding while minimizing lending risk.
Synthetic Data brings technological change in financial systems in three key ways:
ConnectIQ, can synthesize data from scratch or by looking at your existing datasets and uses AI to build data models and automatically create synthetic data for missing data combinations, for data virtualization or excess scenarios on demand at a fraction of the time and cost.
Here’s an example:
A tester might want to test the calculation of the accruals for Different Account Categories per different types of Customers. In this instance the tester might therefore specify criteria based on the Category values of the Category table in order to test the different types of Account opening.
However, the execution of the end-to-end tests cannot be performed using the data only from the Category table. The tester needs to know which Currency to select as well as the Customer. The Customer value has test data which differentiate the customer types based on Sector, Industry, etc.
The data subsets require data from all the parent tables. This is where the data crawling is valuable. The subsetting will automatically work from the criteria specified for the AGC GDI GCI tables, crawling iteratively across all the interrelated tables to collect data related to the subsetted rows.
According to Gartner "By 2024, 60% of the data used for the development of AI analytics projects, will be synthetically generated" and the use of synthetic data allows to deliver quality test data faster significantly reducing project delivery times.
It’s true that in the financial sector you can’t afford to play fast and loose with sensitive data, but the good news is that there is actually a way to keep up with the pace of digital transformation, at a time when market trends are dynamic and unpredictable. For example, synthetic data for financial services enables banks and other financial institutions to build models that vastly improve KYC and customer onboarding while minimizing lending risk.
Synthetic Data brings technological change in financial systems in three key ways:
Enhance privacy of data
As long as your data connects to a real person, there will be always a risk of private information exposure that can’t be eliminated by addressing skills gap in your team or by turning to cloud computing and external providers. On the other hand, scalable privacy-enhancing technologies with a versatile training dataset for machine learning, like synthetic data, ensure that your customers’ data will not slip accidentally or get robbed. As privacy and security legislation intensifies around the world, more financial institutions are exploring how to generate synthetic data for their machine learning projects. The challenge of navigating high volume, complex, potentially unstructured data stored in sprawling databases of silos is solved with synthetic data.Data innovation
Empowering data innovation can help you beat the competition, improve user experience and ensure you meet customer expectations. Without any of the privacy risks of the underlying dataset to worry about, synthetic data is a game changer in the privacy innovation debate. Developers using synthetic data aren’t bound by compliance and regulatory restrictions, allowing them to free up data ‘unlock’ new flexible ways to use data architecture and cloud-based infrastructure to stay agile and boost revenue. This way financial institutions obtain a groundwork for productive collaborations and innovations needed to thrive in the market.Monetize your data
Are you missing out on your data’s potential? New revenue streams can be developed by generating synthetic data based on your production data. A synthetic dataset of aggregate values could be very useful for the retail sector, for example, as in terms of its statistical makeup, is indistinguishable from the real one. Selling original customer data to third parties is restrained because even if you anonymized it, the risk of re-identification will still be there. By using synthetic data you capitalize on a lucrative new revenue-generation opportunity without putting user privacy at risk.Create realistic, privacy-compliant, ‘fit-for-purpose’ data to test your Temenos system
Talking with facts, banks can accelerate test data provisioning times by 95% and shorten the testing and development cycles by several days every sprint. ConnectIQ provides safe, smart and scalable test data with endless variations that allow banks to move beyond data-based challenges. One big asset of synthetic test data set is that it has the same predictive power as the real data, but none of the privacy concerns that impose user restrictions.ConnectIQ, can synthesize data from scratch or by looking at your existing datasets and uses AI to build data models and automatically create synthetic data for missing data combinations, for data virtualization or excess scenarios on demand at a fraction of the time and cost.
Here’s an example:
A tester might want to test the calculation of the accruals for Different Account Categories per different types of Customers. In this instance the tester might therefore specify criteria based on the Category values of the Category table in order to test the different types of Account opening.
However, the execution of the end-to-end tests cannot be performed using the data only from the Category table. The tester needs to know which Currency to select as well as the Customer. The Customer value has test data which differentiate the customer types based on Sector, Industry, etc.
The data subsets require data from all the parent tables. This is where the data crawling is valuable. The subsetting will automatically work from the criteria specified for the AGC GDI GCI tables, crawling iteratively across all the interrelated tables to collect data related to the subsetted rows.
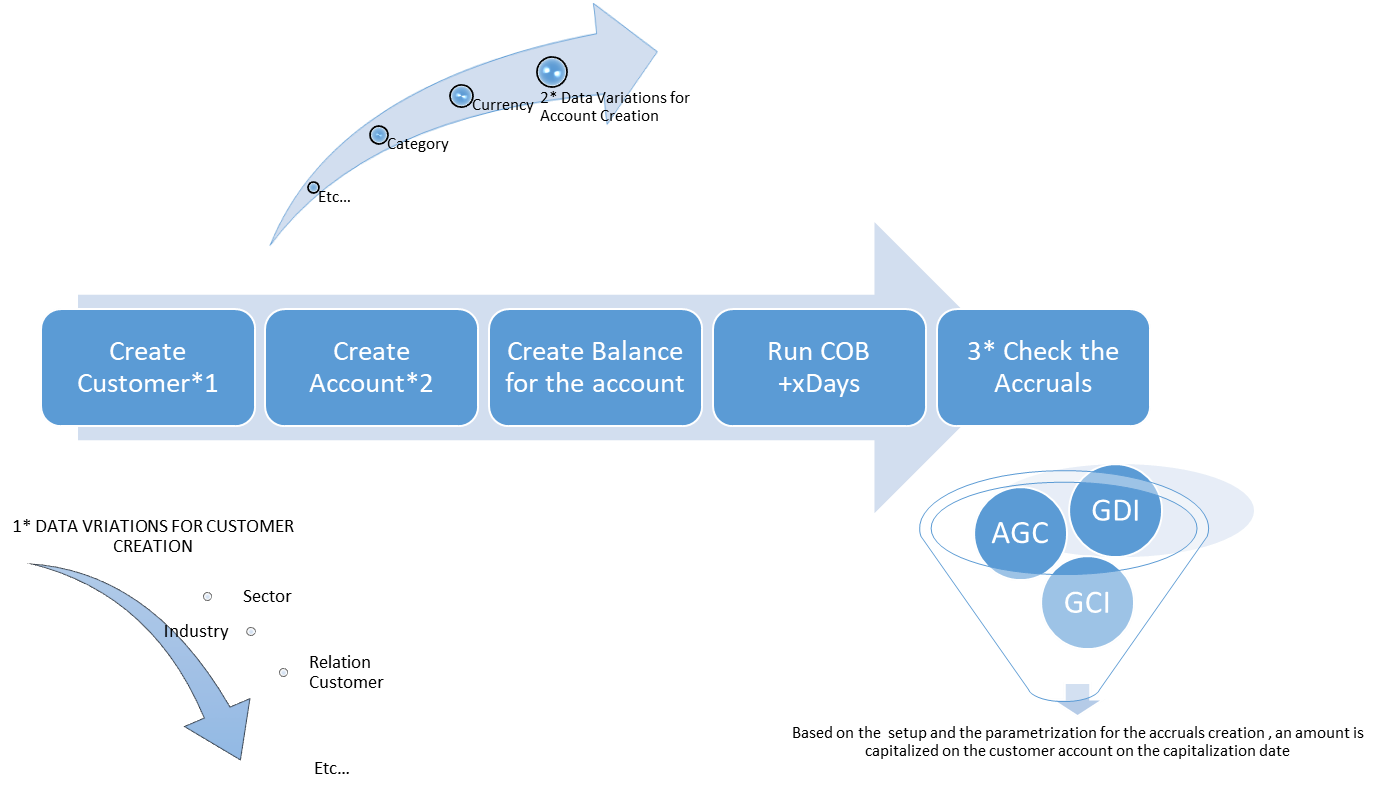
According to Gartner "By 2024, 60% of the data used for the development of AI analytics projects, will be synthetically generated" and the use of synthetic data allows to deliver quality test data faster significantly reducing project delivery times.
Related items
- Modernizing Reconciliation and Investigation for Payment operations
- Transforming Payment Reconciliations and Investigations for improved operational efficiency
- 2023's Top Banking Software Glitches Exposed
- Breaking Free: Why It's Time to Ditch Manual Spreadsheets for Reconciliation
- Leveraging Synthetic Test Data for Software Testing